Abstract
Treatment-resistant depression is a severe form of major depressive disorder and deep brain stimulation is currently an investigational treatment. The stimulation’s therapeutic effect may be explained through the functional and structural connectivities between the stimulated area and other brain regions, or to depression-associated networks. In this longitudinal, retrospective study, four female patients with treatment-resistant depression were implanted for stimulation in the nucleus accumbens area at our center. We analyzed the structural and functional connectivity of the stimulation area: the structural connectivity was investigated with probabilistic tractography; the functional connectivity was estimated by combining patient-specific stimulation volumes and a normative functional connectome. These structural and functional connectivity profiles were then related to four clinical outcome scores. At 1-year follow-up, the remission rate was 66%. We observed a consistent structural connectivity to Brodmann area 25 in the patient with the longest remission phase. The functional connectivity analysis resulted in patient-specific R-maps describing brain areas significantly correlated with symptom improvement in this patient, notably the prefrontal cortex. But the connectivity analysis was mixed across patients, calling for confirmation in a larger cohort and over longer time periods.
Similar content being viewed by others
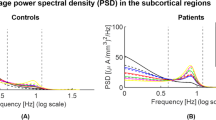
Introduction
Major depressive disorder (MDD) is characterized by depressed mood or loss of interest, and often presents a chronic course [1]. About a third [2] of affected patients do not respond adequately to conventional pharmaceutical or cognitive behavioral therapy [3, 4] and develop treatment-resistant depression (TRD), a particularly debilitating subcategory of MDD [5].
Depressive symptoms have been associated with pathological connectivity in at least four brain subnetworks: the ventral limbic affective network, the dorsal cognitive control network, the default mode network, and the frontal–striatal reward network [6,7,8]. The dysregulation of the reward network has been specifically related to anhedonia (i.e., loss of interest, motivation, and pleasure) [6, 9,10,11,12].
Deep brain stimulation (DBS) is being investigated as treatment option for TRD patients [10, 13,14,15]. The antidepressant effect of DBS depends on the neuroanatomical target, the stimulation parameters, and the modulation of both the target and the connected networks [16]. Several stimulation targets have been proposed: the subcallosal cingulate gyrus [13], the medial forebrain bundle [17], the ventral capsule/ventral striatum or anterior limb of internal capsule, the lateral habenula, the inferior thalamic peduncle, and the nucleus accumbens (NAc) [16, 18].
These targets presumably improve depressive symptoms by modulating a common network [19]. A recent study [20] suggests that not only different DBS targets, but also transcranial magnetic stimulation targets and brain lesions are interconnected and converge on a common depression network. Likewise, different DBS targets for obsessive–compulsive disorder, a disorder closely related to TRD [17, 21], converge on one network [22,23,24]. Still, it is essential to differentiate the impact of each DBS target and to analyze longitudinal outcome data to inform more effective DBS for TRD [25, 26].
The NAc has been investigated as a target because of its central role in the reward circuitry [27]. Structurally, the medial forebrain bundle connects the NAc to the ventral tegmental area, the ventromedial hypothalamus, the lateral hypothalamus, and the amygdala, with convergence onto the prefrontal cortex [28, 29], all crucial regions in the pathobiology of depression [6]. In particular, microstructural white-matter alterations of the medial forebrain bundle have been associated with symptoms of anhedonia [11]. Functionally, the serotonin–dopamine–glutamate interactions occurring in the NAc also make it a candidate target for DBS for MDD. Moreover, modulation of this area might restore the equilibrium between inhibitory and excitatory neurotransmission necessary for reward processing and mood regulation [27].
NAc gray-matter alterations have been associated with MDD symptoms and lifetime occurrence of the disease [30]. Additionally, reduced responses to rewarding stimuli in the NAc have been reported in patients with MDD [27, 31]; and anhedonia has been correlated with functional connectivity of specific NAc subregions [32]. Finally, clinical and neurobiological studies demonstrated that DBS of the NAc area reduces anhedonia [14, 33, 34].
The objective of the current study was to correlate clinical improvement related to DBS of the NAc area with the area’s structural and functional connectivity, measured through diffusion MRI (dMRI) and functional MRI (fMRI). We hypothesized a correlation between DBS connectivity to the reward network and clinical improvement. Furthermore, we expected beneficial NAc area DBS to be functionally and/or structurally connected to other nodes of the depression network.
Materials and methods
Patients and data
Four patients with TRD from the University Hospital Bern (Bern, Switzerland) were initially included in this retrospective study. The local ethics committee approved the study (2020-02392), and patient consent was obtained prior to the treatment. After DBS implantation, the patients agreed to have their data used in this post hoc analysis. Such consent was given to the neurology department during their standard pre-surgical workup. Patients 1, 3, and 4 were diagnosed with recurrent MDD, while patient 2 had bipolar disorder with predominantly depressive episodes. None of the patients had medical or neurological comorbidities. All underwent cycles of standard antidepressants (more than three, including tricyclics), lithium, ketamine, specific psychotherapy interventions for TRD [cognitive behavioral therapy and cognitive behavioral analysis system of psychotherapy (CBASP)], and electro-convulsive therapy prior to surgery, yet failed to reach remission. The study participants were the only patients who received bilateral DBS surgery for depression at our center between 2017 and 2020.
Targeting of the NAc area was performed on structural MRI and dMRI sequences with tractography of the medial forebrain bundle. The target was placed just posterior to the NAc in order to include the afferent fibers of the medial forebrain bundle before reaching the anterior limb of the internal capsule (Supplementary Fig. 1). Table 1 reports the diagnosis, sex, age, and baseline depression scores of the patients at the time of implantation. Patient 4 was excluded from the rest of the analysis due to the lack of patient-specific dMRI.
Clinical assessments
Patients were routinely assessed after surgery with weekly sessions initially that became less frequent afterwards. Four standardized MDD assessments were adopted: HAMD-21 (21-Item Hamilton Depression Rating Scale) [35], MADRS (Montgomery–Asberg Depression Rating Scale) [36], SHAPS (Snaith–Hamilton Pleasure Scale) [37], and SOFAS (Social and Occupational Functioning Assessment Scale) [38]. The SHAPS scale specifically describes anhedonia and is appropriate to describe the modulation of the NAc area because of this structure’s role in the reward system [39]. The traditionally used HAMD-21 scale is unbalanced towards sleep and circadian dysregulations [40, 41], while the MADRS is considered to be well balanced across the different MDD somatic and cognitive symptoms [42]. Psychiatric assessments were completed by LS, FW, CS, and MS, senior psychiatrists and clinical psychologists with extensive experience and training in assessing psychopathology, including the HAMD and MADRS. They were supervised by SW.
To ease comparison, all scales were normalized onto a scale of 0–100, with high values indicating severe depression. The percentage improvement with respect to baseline was recorded at each clinical assessment and considered as the effect of the most recent DBS parameters applied. The stimulation parameters were fine-tuned to minimize the symptoms and achieve remission (HAMD-21 < 8) or at least response (> 50% improvement of the HAMD-21 score from baseline). The follow-up period was at least 18 months but variable across patients. The number of assessment sessions was 36 for patient 1, 11 for patient 2, and 14 for patient 3, respectively. In each session, the ongoing DBS parameters were recorded.
Patient-specific imaging
Pre-implantation neuroimaging was performed on a 3T scanner (Magnetom Skyra Fit, Siemens, Germany) using a 32-channel head receiver coil. The protocol included T1-weighted (TR = 2020 ms, TE = 3.49 ms, voxel resolution 1 × 1 × 1 mm3) and T2-weighted (TR = 2400 ms, TE = 225 ms, voxel resolution 1 × 1 × 1 mm3) sequences, and a dMRI sequence acquired in the Siemens q-space mode (repetition time = 5900 ms, echo time = 111 ms, voxel resolution 2.2 × 2.2 × 2.2 mm3, in-plane acceleration GRAPPA factor of 2, partial Fourier 7/8, field of view 211 × 211 mm2). Diffusion weighting, with b-values in the range of 0–3000 s/mm2 was applied along 123 directions uniformly distributed on the sphere. Postoperative CT or MRI scans were also acquired.
Functional imaging
Minimally pre-processed resting-state fMRI acquisitions of 30 random healthy subjects (15 females) from the Human Connectome Project (HCP) database were included to build a reference functional connectome (Supplementary Table 1) [43]. While our clinical sample differed in age and consisted exclusively of females, our random selection of HCP subjects was driven by practical and scientific considerations. The HCP data represents a widely utilized neuroimaging database, including young adults aged 22–35. Furthermore, as TRD can affect both genders, opting for a sex-balanced dataset was justified. Although a larger sample size might have been useful, we limited our sample size to 30 subjects due to computational constraints (analysis took up to 3 days per subject).
The resting-state data consisted of four sessions of approximately 15 min each. Acquisition parameters are described in (https://db.humanconnectome.org). Further pre-processing was performed as in [44], including spatial smoothing and remotion of the first ten volumes, detrending, regressing of nuisance variables (head translation and rotation along the three axes, average cerebrospinal fluid, and white-matter signal) and band-pass filtering [0.01–0.15 Hz]. To avoid well-known artifactual negative correlations induced by global signal regression and the removal of signal of neural origin that might be of interest for the analysis [45, 46], we chose here to be conservative and not perform global signal regression. We obtained a V-by-T data matrix for each subject containing the pre-processed BOLD time-courses, with V the number of voxels and T the number of timepoints. The four sessions were then concatenated temporally to obtain a V-by-4T matrix for each subject [47].
Lead localization and volume of activated tissue (VAT) estimation
Lead localization was performed with the Lead-DBS toolbox (version 2.5.2) [48] in MATLAB 2020b (The Mathworks, Natick, MA, USA) (Supplementary Fig. 2). Using an early postoperative high-resolution CT or MRI scan, DBS leads were semi-automatically localized either with the PaCER [49] or with the TRAC/CORE [50] algorithm (postoperative CT or MRI case, respectively). For postoperative CTs, the orientation was determined with DiODe [51]. For postoperative MRIs, the orientation was determined with early postoperative X-rays.
Volumes of Activated Tissue (VATs) were estimated with the SimBio/Fieldtrip pipeline in Lead-DBS with default parameters for the conductivity of gray and white matter and electric field threshold [52]. During the clinical assessments, stimulation parameters for each DBS lead were recorded, including the contact and stimulation intensity. These parameters (Supplementary Table 2) were used to generate the corresponding VATs (Fig. 1A). The VATs were computed for the left and right leads. In total, there were 122 VATs or 61 bilateral VATs.
Structural connectivity analysis pipeline. A Lead localization in patient space and generation of the bilateral VAT (red volumes) based on individual stimulation parameters. B Generation of patient-specific whole-brain tractogram. C Selection of streamlines included in the bilateral VAT. D Assignment of streamlines’ endpoints to a Brodmann and subcortical parcellation. VAT volume of activated tissue
DBS connectivity
Structural connectivity
The structural connectivity analysis was performed in MRtrix3 (https://www.mrtrix.org/) [53]. Preprocessing steps included: denoising [54], unringing [55], distortion correction with the Synb0-DisCo tool [56], eddy-currents and subject movement correction [57]. To obtain whole-brain probabilistic tractography, we first estimated the individual multi-shell multi-tissue response functions [58]. Based on the population-averaged response functions, we derived the patient-specific fiber orientation distribution through constrained spherical deconvolution [59] and performed intensity normalization [60]. Next, using anatomically constrained tractography [61] we generated 10 million streamlines (Fig. 1B). Finally, by applying the filtering algorithm SIFT2 [62], each streamline got a weight assigned. Since the SIFT2 algorithm considers all generated streamlines, it provides the tractogram with quantitative properties [63] and is, thus, a more accurate metric of structural connectivity than the streamlines’ count [64] or voxel-based structural connectivity approaches [65].
From each patient’s whole-brain tractogram, we selected only the streamlines passing through the bilateral VAT (Fig. 1C). Next, we identified the end points of these streamlines and assigned them to a parcellation containing cortical and subcortical structures (Fig. 1D). Cortical areas were defined with the Broadman parcellation [66], and subcortical areas (including NAc area, amygdala, cerebellum, hippocampus, pallidum, putamen, and thalamus) were defined with FreeSurfer (https://surfer.nmr.mgh.harvard.edu/). Streamlines whose end points were not in any of the defined areas were discarded.
The structural connectivity strength between a VAT and a parcellation area was measured as the sum of the weights of the streamlines that connected the VAT with that area and normalized to the total sum of weights of the streamlines in that VAT. We calculated the structural connectivity strength profile for each VAT, as expressed by Eq. (1):
Finally, the structural connectivity strengths of left and right VATs were combined. As the effect of unilateral stimulation was not evaluated during the assessment sessions, we considered the bilateral VAT from the same session as a single stimulated volume and combined bilateral areas of the parcellation.
Functional connectivity
We computed the seed-to-voxel functional connectivity similar to [22, 65]. In particular, we used the fMRI data from 30 Human Connectome Project (HCP) subjects and the patient-specific bilateral VATs as regions of interest. For each clinical assessment and the corresponding bilateral VAT, we performed the following steps to generate the functional connectivity profile, in which each voxel represented the functional connectivity strength between the bilateral VAT and the considered target voxel (A).
-
1.
Average the activity across all voxels of a bilateral VAT.
-
2.
Compute the Pearson’s correlation coefficient between a bilateral VAT’s average activity and each non-VAT voxel.
-
3.
Repeat step (2) for each of the 30 HCP subjects.
-
4.
For each voxel, average the Pearson’s correlation coefficient across the 30 HCP subjects.
-
5.
Apply Fisher z-transform to obtain a normal distribution of Pearson’s correlation coefficients, to yield a VAT-specific population-averaged connectivity map, what we call functional connectivity profile.
The connectivity profile was coupled with the clinical assessment to derive a patient-specific, voxel-wise connectivity R-map, as described in the following example with the SHAPS scale (Fig. 2B).
Functional connectivity analysis pipeline. A VAT reconstruction and creation of VAT-specific functional connectivity profile. Unilateral, rather than bilateral, VAT is shown for simplicity. B Creation of a patient-specific R-map describing the relation between functional connectivity and symptoms’ improvement. Adapted, with permission, from [22]. VAT volume of activated tissue, HCP Human Connectome Project
First, we obtained the vector of SHAPS improvement with respect to baseline across clinical assessment sessions for a patient. Since each session corresponds to a functional connectivity profile, we considered all the patient’s functional profiles and, for each voxel, stored its bilateral VAT connectivity across sessions. The correlation between every voxel connectivity vector and the vector of SHAPS score changes resulted in an R-map. The procedure, adopted from [22], was used to obtain an R-map for all MDD assessment scales. These personalized R-maps are spatial indicators of “optimal” bilateral VAT functional connectivity: positive voxels indicate areas that, when functionally connected to the bilateral VAT, relate to improvement as measured in the corresponding scale. The opposite holds for negative voxels.
Statistics
For structural connectivity, we obtained structural connectivity strengths, or profiles. Then, we computed the Spearman’s correlation coefficients between the connectivity strengths to individual areas in the parcellation and the assessment scores. We repeated the analysis for each MDD scale used. We performed this correlation analysis for the three patients individually. We used false discovery rate-adjusted p-values to determine the statistically significant structural connections. The correlation analysis and false discovery rate corrections were performed in Python (version 3.9.2) with the scipy (version 1.9) and statsmodels (version 0.13.2) libraries, respectively.
Likewise for functional connectivity, we obtained functional connectivity strengths, or profiles. Then we tested their association with clinical outcomes by computing patient-specific R-maps. To test the statistical significance of the R-maps, we adopted the non-parametric permutation test with custom code in MATLAB 2020b as described in [67] and detailed in the supplementary material.
Results
Longitudinal analysis
The follow-up period was at least 18 months in all cases and contained the assessments of the HAMD-21, MADRS, SHAPS, and SOFAS scales. One year after implantation, 2 out of 3 patients were in remission.
For patient 1, an improvement was noticeable at 2.5 months post-implantation (session 10), especially on the SHAPS scale. From 9 months post-implantation (session 25), the improvement was observed across all scales, corresponding to a prolonged remission period of 10 months. In the last three sessions, a worsening on the HAMD scale occurred, marking the end of remission (Fig. 3A).
Longitudinal analysis of clinical scales and structural connectivity strengths. A Course of clinical rating scale scores. Clinical improvement was obtained as the percentage of improvement with respect to the baseline. HAMD-21 21-Item Hamilton Depression Rating Scale, MADRS Montgomery–Asberg Depression Rating Scale, SHAPS Snaith–Hamilton Pleasure Scale, and SOFAS Social and Occupational Functioning Assessment Scale. Remission state is indicated with ⧫. B Bilateral VATs structural connectivity profiles to Brodmann areas (1–47) and subcortical structures (nucleus accumbens, amygdala, cerebellum, hippocampus, pallidum, putamen, and thalamus). Each column represents the connectivity from a bilateral VAT to the different parcellation areas. Bilateral VATs were estimated from the stimulation settings at each of the assessment sessions. VAT volume of activated tissue
For patients 2 and 3, a similarly strong improvement across scales was not observed. But for these patients the number of assessments was lower, covering a period of 19 and 26 months, respectively. For patient 3, a remission period of 16 months started at 9 months post-implantation (session 11), with improvements on the MADRS and SOFAS scales. Improvements in the SHAPS scales occurred from month 22 post-implantation (session 13).
Structural connectivity
First, we analyzed the structural connectivity over the follow-up period. The three patients’ structural connectivity profiles from the bilateral VATs to the Brodmann Areas (BAs) shared, with different intensities, strong cortical connections to emotional (BA 11, 38, 47), attentional (BA 7), visual (BA 17–19), memory (BA 20, 35, 36, 37) and executive (BA 10) areas (Fig. 3B). In patient 1, we observed a strong connectivity to the subgenual anterior cingulate cortex (BA 25) and the temporopolar area (BA 38). The connectivity to these areas was stable across the bilateral VATs, with a median value of 10.4% (Inter-quartile range, IQR 9.8–11.5) for BA 25 and 8.2% (IQR 8.1–8.3) for BA 38. In patients 2 and 3, a strong connectivity to BA 25 was not observed, but the median connectivity to BA 38 was 3.3% (IQR 2.7–4.3) and 2.7% (IQR 2.3–2.7), respectively (Fig. 3B). In these two patients, we also observed enhanced connectivity to the prefrontal cortex (BA 10) and orbitofrontal area (BA11) compared to patient 1, but less than for the previously mentioned areas.
Structural connectivity to the subcortical structures was also relevant (Fig. 3B). The three patients shared a strong connectivity to the thalamus, putamen and accumbens area, while patients 2 and 3 also had a strong connectivity to the pallidum. The median thalamic connectivity of patient 1 (23.2%, IQR 22.9–24) was more stable across the bilateral VATs and stronger than for patient 2 (22.2%, IQR 17.9–25.6) or patient 3 (17.2%, IQR 15.5–21.2).
Second, we analyzed correlations between the structural connectivity and the clinical outcome. In patient 1, structural connectivity to the motor (BA 32), executive (BA 10 and 44), and emotional (BA 11 and 47) cortices was positively correlated with improved outcomes (Fig. 4). Connectivity to the amygdala, cerebellum, hippocampus, and putamen was also positively correlated with improved outcomes. In contrast, in patients 2 and 3, the structural connectivity was not significantly correlated with outcome (Fig. 4).
Spearman’s correlations between the assessment scores and the structural connectivity strength from the bilateral VATs to the parcellation areas. Correlation coefficients were calculated for each patient individually. Significant correlations after correcting for type I errors with false discovery rate are marked with an asterisk. Brodmann areas in the parcellation (1–47) are grouped into functional areas (e.g., somatosensory, motor, executive, emotional, insular, attention, memory, visual, olfactory, sound). VAT volume of activated tissue
Functional connectivity
For patient 1, some areas demonstrated significant correlations between functional connectivity and symptoms improvement (Fig. 5). The red areas in Fig. 5, corresponding mainly to executive areas (BA 9 and 10), were correlated with improvement on the SHAPS and MADRS scales. Conversely, blue areas were negatively correlated to improvement on these two scales. These mainly corresponded to visual (BA 18 and 19) and attentional areas (BA 7). Across scales, the areas of positive and negative correlation to improvement were similar in location, but the size of the areas varied.
Functional connectivity R-maps of patient 1, visualized with BrainNet Viewer [88]. A SHAPS (Snaith–Hamilton Pleasure Scale); B MADRS (Montgomery–Asberg Depression Rating Scale)
The in-depth voxel-wise analysis of the significant R-maps is shown in Supplementary Tables 3 and 4. The functional connectivity analyses of patients 2 and 3 were non-significant. For these patients, no brain area was significantly correlated with improvement on any of the scales.
Discussion
In this retrospective case series of TRD patients implanted with DBS in the NAc, no serious adverse events were noted. The structural and functional connectivity profiles of the VATs were mixed across patients.
Structural connectivity
Our study highlighted a structural connectivity of the bilateral VATs to the subgenual anterior cingulate cortex (BA 25) in patient 1, who had the longest remission period. This connection was expected from both dissection [68] and tractography studies [69]. BA 25 has been implicated in persistent sad mood [70], circadian dysregulations [13] and rumination [71] and is a key node of the affective network [6]. This area is metabolically hyperactive in MDD patients [72], and successful treatment through medication (e.g., fluoxetine, venlafaxine), electro-convulsive therapy, cognitive behavioral therapy, and repetitive transcranial magnetic stimulation has been associated with a reduction in its activity [73, 74]. Interestingly, for patients 2 and 3, the structural connectivity to BA 25 was less prominent and coincided with less clinical improvements.
As in [13, 70, 75], we hypothesized a correlation between this area’s connectivity to the bilateral VATs and clinical improvement. In patient 1, the structural connectivity was strongest to BA 25 and it was stable across sessions. In this subject, structural connectivity to this area was positively correlated to the outcome, but was statistically non-significant. The limited intrasubject changes in BA 25 connectivity could derive from the very few changes in the DBS contact selection and from the time-invariance assumption of the whole-brain tractogram (i.e., assuming the tractogram does not change with implantation nor over time).
Riva-Posse et al. [76] identified four bundles whose activation was correlated with clinical response to DBS in BA 25 for TRD: bilateral forceps minor, bilateral cingulum bundles, dorsal and medial midcingulate cortices, and uncinate fasciculus. The uncinate fasciculus interconnects the lateral orbitofrontal cortex and BA10 to the limbic regions of the anterior temporal lobes. The structural connectivity of our best responder (patient 1) also highlighted connection to the temporopolar area (BA 38). The structural connectivity between our target site (i.e., NAc area) and the temporopolar area suggests activation of the uncinate fasciculus bundle, thus agreeing with [76]. The structural connectivity profiles of patients 2 and 3 also showed connectivity to BA 38; but in the correlation analysis, connectivity to this area was not positively correlated with clinical improvement.
GABA-ergic and dopaminergic connections between the NAc, amygdala, and hippocampus seem to be related to the regulation of emotional responses [27, 77]. In our best responder, patient 1, we observed a higher structural connectivity to the amygdala and hippocampus; and in the correlation analysis, these areas were positively correlated with the outcome. This finding in patient 1 is in line with a recent DBS study using network mapping and biomarker discovery, which also highlighted the connectivity to the amygdala as an indicator of symptom improvement [77].
Functional connectivity
The optimal connectivity maps obtained allowed us to relate the bilateral VATs’ functional connectivity to the outcomes. The cortical areas most strongly correlated to a positive outcome were all belonging to the prefrontal cortex (BA 8, 9, 10, 24, 32, 45, 46). The prefrontal cortex is thought to give rise to the psychomotor and cognitive aspects of TRD [78, 79] and is a commonly used repetitive transcranial magnetic stimulation target for TRD [80]. In the functional connectivity maps, BA 25 either showed an average correlation coefficient close to zero or had a low number of significant voxels. This agrees with our structural connectivity analysis that BA 25 is structurally connected to the bilateral VATs irrespective of the outcome. For patients 2 and 3, there was no significant voxel after the permutation test. This might have a twofold explanation. First, these patients only had 11 and 14 assessment sessions, respectively, while patient 1 had 36. Besides, the sessions of patients 2 and 3 did not show a large variability in outcomes. Therefore, the creation of functional connectivity maps may crucially depend on the availability of larger and more variable patient outcome data.
Nucleus accumbens area as a DBS target
Our study expands the literature on the NAc as DBS target. The NAc seems to be at the center of a limbic corticostriatal loop, between the emotional system, the cognitive system and the motor control system [74]. Given the area’s cortical and subcortical connections, we included both in the parcellation used to study the structural connectivity profiles of DBS. Because of its role in the reward network [81], the NAc is an established DBS target for TRD and it is especially suited for depression types with prevailing anhedonia [25].
Our response rate was in line with the open-label NAc DBS studies of Bewernick et al. [33, 34], which reported a 50% response rate and a 30% remission rate at 1-year follow-up, and 45.5% and 9.1% at 2-year follow-up. These response rates were comparable to those of other DBS targets for TRD such as the medial forebrain bundle [82, 83], the subgenual anterior cingulate cortex target [84, 85], and the inferior thalamic peduncle [86, 87].
The improvements observed in our best responder may have a twofold explanation. On the one hand, there was structural and functional connectivity from the VATs to nodes of the depression network such as BA 25, the thalamus, amygdala, or the prefrontal cortex. On the other hand, the cortical connectivity of our target site suggests a possible overlap with the medial forebrain bundle or ventral tegmental area projection pathway, which has been proposed as a DBS target [88]. The tract is highly similar to the NAc crossing bundle described by [23], which has been related to improvement for obsessive–compulsive disorder, a condition sharing many features with MDD. Since single-target clinical studies failed to prove efficacy, we might argue, as in [19, 76, 89], that the stimulation of a unique target is insufficient for remission, and future protocols should aim to engage the distributed depression network instead.
Study limitations
The present work has several limitations. First, it is restricted to three subjects, some assessed in few sessions. The study was open label and did not have standardized time intervals during long-term follow-ups. The stimulation parameters were changed based on the previous symptom’s evolution in a trial-and-error fashion, potentially introducing a placebo effect. However, typically, the placebo effects are less pronounced in subjects with severe or chronic depression [90].
Second, our analysis did not consider medications or external life events. These are likely to affect the clinical outcome but may not be reflected in the connectivity profiles or R-maps. We acknowledge that the assumption of DBS as the unique or main source of clinical outcome is a simplification.
Lastly, we used fMRI data from the Human Connectome Project, rather than patient-specific fMRI, to create functional connectivity R-maps. Normative connectomes have been leveraged for the study of many psychological conditions. In the DBS field, the use of normative data has been a key to identify patterns of beneficial connectivity [22, 65]. While the patient data should be more accurate in describing pathological connections, a recent study suggests that the difference is minimal and counterbalanced by the quality of large datasets such as the Human Connectome Project [91].
Conclusions and outlook
We have leveraged patient-specific diffusion images and probabilistic tractography to quantify the structural connectivity of NAc area DBS to cortical and subcortical brain areas. The subgenual anterior cingulate cortex (BA 25), known for its involvement in depression, was consistently and strongly connected to bilateral VATs in the patient experiencing extended periods of remission. This suggests that effective NAc area DBS for TRD may modulate a network shared also with other DBS targets.
We created statistically significant R-maps associating the bilateral VATs’ functional connectivity and improvements. Their analysis revealed that connectivity to the prefrontal cortex was associated with improvement in one patient. Once validated with more MRI data and statistical tests, these maps could have important clinical applications and could suggest stimulation parameters, thus becoming valuable features for personalized closed-loop DBS [92]. Furthermore, they could guide complementary TRD therapies that would only engage areas linked to improvement.
Our work adopts the much needed [19] patient-centric approach in the description of DBS connectivity. Overall, the results were variable among the three patients and could only partially describe the observed clinical TRD progression. Further studies, including more patients over longer time periods, are needed to better understand the connectivity of effective DBS for TRD. In particular, we encourage prospective NAc DBS studies to adopt blinded multidimensional assessment of MDD symptoms, including longitudinal ambulatory assessments using ecological momentary assessments, and to include other neuroimaging data, e.g., fMRI and EEG, repeated over multiple sessions.
Data availability
Pipelines for dMRI processing and data analysis are available in: https://gitlab.switch.ch/brain-stimulation-mapping/2025_dbsinmdd. This is a preliminary repository, and the name will be updated upon publication. A de-identified data set containing tracts in patient space, the MNI VTAs and transformations from MNI to patient space can be provided on application, subject to institutional review board approval.
References
Kupfer DJ, Frank E, Phillips ML (2012) Major depressive disorder: new clinical, neurobiological, and treatment perspectives. Lancet 379:1045–1055
Rush AJ, Trivedi MH, Wisniewski SR, Nierenberg AA, Stewart JW, Warden D et al (2006) Acute and longer-term outcomes in depressed outpatients requiring one or several treatment steps: a STAR* D report. Am J Psychiatry 163:1905–1917
Fava M (2003) Diagnosis and definition of treatment-resistant depression. Biol Psychiatry 53:649–659
Sforzini L, Worrell C, Kose M, Anderson IM, Aouizerate B, Arolt V et al (2022) A Delphi-method-based consensus guideline for definition of treatment-resistant depression for clinical trials [no. 3]. Mol Psychiatry 27:1286–1299
Mrazek DA, Hornberger JC, Altar CA, Degtiar I (2014) A review of the clinical, economic, and societal burden of treatment-resistant depression: 1996–2013. Psychiatr Serv 65:977–987
Li B-J, Friston K, Mody M, Wang H-N, Lu H-B, Hu D-W (2018) A brain network model for depression: from symptom understanding to disease intervention. CNS Neurosci Ther 24:1004–1019
Korgaonkar MS, Fornito A, Williams LM, Grieve SM (2014) Abnormal structural networks characterize major depressive disorder: a connectome analysis. Biol Psychiatry 76:567–574
Williams LM (2016) Precision psychiatry: a neural circuit taxonomy for depression and anxiety. Lancet Psychiatry 3:472–480
Russo SJ, Nestler EJ (2013) The brain reward circuitry in mood disorders [no. 9]. Nat Rev Neurosci 14:609–625
Fenoy AJ, Quevedo J, Soares JC (2022) Deep brain stimulation of the “medial forebrain bundle”: a strategy to modulate the reward system and manage treatment-resistant depression [no. 1]. Mol Psychiatry 27:574–592
Bracht T, Horn H, Strik W, Federspiel A, Schnell S, Höfle O et al (2014) White matter microstructure alterations of the medial forebrain bundle in melancholic depression. J Affect Disord 155:186–193
Bracht T, Mertse N, Walther S, Lüdi K, Breit S, Federspiel A et al (2022) Link between structural connectivity of the medial forebrain bundle, functional connectivity of the ventral tegmental area, and anhedonia in unipolar depression. NeuroImage Clin 34:102961
Mayberg HS, Lozano AM, Voon V, McNeely HE, Seminowicz D, Hamani C et al (2005) Deep brain stimulation for treatment-resistant depression. Neuron 45:651–660
Schlaepfer TE, Bewernick BH, Kayser S, Mädler B, Coenen VA (2013) Rapid effects of deep brain stimulation for treatment-resistant major depression. Biol Psychiatry 73:1204–1212
Zhu Z, Hubbard E, Guo X, Barbosa DAN, Popal AM, Cai C et al (2021) A connectomic analysis of deep brain stimulation for treatment-resistant depression. Brain Stimul 14:1226–1233
Dandekar MP, Fenoy AJ, Carvalho AF, Soares JC, Quevedo J (2018) Deep brain stimulation for treatment-resistant depression: an integrative review of preclinical and clinical findings and translational implications [no. 5]. Mol Psychiatry 23:1094–1112
Coenen VA, Schlaepfer TE, Goll P, Reinacher PC, Voderholzer U, Tebartz van Elst L et al (2017) The medial forebrain bundle as a target for deep brain stimulation for obsessive-compulsive disorder. CNS Spectr 22:282–289
Drobisz D, Damborská A (2019) Deep brain stimulation targets for treating depression. Behav Brain Res 359:266–273
Schlaepfer TE, Bewernick BH, Kayser S, Hurlemann R, Coenen VA (2014) Deep brain stimulation of the human reward system for major depression—rationale, outcomes and outlook [no. 6]. Neuropsychopharmacology 39:1303–1314
Siddiqi SH, Schaper FLWVJ, Horn A, Hsu J, Padmanabhan JL, Brodtmann A et al (2021) Brain stimulation and brain lesions converge on common causal circuits in neuropsychiatric disease [no. 12]. Nat Hum Behav 5:1707–1716
Bolhuis K, McAdams T, Monzani B, Gregory AM, Mataix-Cols D, Stringaris A, Eley TC (2014) Aetiological overlap between obsessive–compulsive and depressive symptoms: a longitudinal twin study in adolescents and adults. Psychol Med 44:1439–1449
Li N, Hollunder B, Baldermann JC, Kibleur A, Treu S, Akram H et al (2021) A unified functional network target for deep brain stimulation in obsessive–compulsive disorder. Biol Psychiatry 90:701–713
Li N, Baldermann JC, Kibleur A, Treu S, Akram H, Elias GJB et al (2020) A unified connectomic target for deep brain stimulation in obsessive-compulsive disorder [no. 1]. Nat Commun 11:3364
Menchón JM, Real E, Alonso P, Aparicio MA, Segalas C, Plans G et al (2021) A prospective international multi-center study on safety and efficacy of deep brain stimulation for resistant obsessive-compulsive disorder [no. 4]. Mol Psychiatry 26:1234–1247
Figee M, Mayberg H (2021) The future of personalized brain stimulation. Nat Med 27:196–197
Hollunder B, Rajamani N, Siddiqi SH, Finke C, Kühn AA, Mayberg HS et al (2022) Toward personalized medicine in connectomic deep brain stimulation. Prog Neurobiol 210:102211
Nauczyciel C, Robic S, Dondaine T, Verin M, Robert G, Drapier D et al (2013) The nucleus accumbens: a target for deep brain stimulation in resistant major depressive disorder. J Mol Psychiatry 1:17
Gálvez JF, Keser Z, Mwangi B, Ghouse AA, Fenoy AJ, Schulz PE et al (2015) The medial forebrain bundle as a deep brain stimulation target for treatment resistant depression: a review of published data. Prog Neuropsychopharmacol Biol Psychiatry 58:59–70
Roet M, Boonstra J, Sahin E, Mulders AE, Leentjens AF, Jahanshahi A (2020) Deep brain stimulation for treatment-resistant depression: towards a more personalized treatment approach. J Clin Med 9:2729
Ancelin M-L, Carrière I, Artero S, Maller J, Meslin C, Ritchie K et al (2019) Lifetime major depression and grey-matter volume. J Psychiatry Neurosci 44:45–53
Pizzagalli DA, Holmes AJ, Dillon DG, Goetz EL, Birk JL, Bogdan R et al (2009) Reduced caudate and nucleus accumbens response to rewards in unmedicated individuals with major depressive disorder. Am J Psychiatry 166:702–710
Liu R, Wang Y, Chen X, Zhang Z, Xiao L, Zhou Y (2021) Anhedonia correlates with functional connectivity of the nucleus accumbens subregions in patients with major depressive disorder. NeuroImage Clin 30:102599
Bewernick BH, Hurlemann R, Matusch A, Kayser S, Grubert C, Hadrysiewicz B et al (2010) Nucleus accumbens deep brain stimulation decreases ratings of depression and anxiety in treatment-resistant depression. Biol Psychiatry 67:110–116
Bewernick BH, Kayser S, Sturm V, Schlaepfer TE (2012) Long-term effects of nucleus accumbens deep brain stimulation in treatment-resistant depression: evidence for sustained efficacy. Neuropsychopharmacology 37:1975–1985
Hamilton M (1967) Development of a rating scale for primary depressive illness. Br J Soc Clin Psychol 6:278–296
Montgomery SA, Åsberg M (1979) A new depression scale designed to be sensitive to change. Br J Psychiatry 134:382–389
Snaith RP, Hamilton M, Morley S, Humayan A, Hargreaves D, Trigwell P (1995) A scale for the assessment of hedonic tone the Snaith–Hamilton pleasure scale. Br J Psychiatry 167:99–103
Rybarczyk B (2011) Social and occupational functioning assessment scale (SOFAS). LXIII Encycl Clin Neuropsychol 1:2313
Fenoy AJ, Schulz P, Selvaraj S, Burrows C, Spiker D, Cao B et al (2016) Deep brain stimulation of the medial forebrain bundle: distinctive responses in resistant depression. J Affect Disord 203:143–151
Demyttenaere K, Kiekens G, Bruffaerts R, Mortier P, Gorwood P, Martin L, Di Giannantonio M (2021) Outcome in depression (I): why symptomatic remission is not good enough. CNS Spectr 26:393–399
Bagby RM, Ryder AG, Schuller DR, Marshall MB (2004) The Hamilton depression rating scale: has the gold standard become a lead weight? Am J Psychiatry 161:2163–2177
Uher R, Farmer A, Maier W, Rietschel M, Hauser J, Marusic A et al (2008) Measuring depression: comparison and integration of three scales in the GENDEP study. Psychol Med 38:289–300
Glasser MF, Sotiropoulos SN, Wilson JA, Coalson TS, Fischl B, Andersson JL et al (2013) The minimal preprocessing pipelines for the Human Connectome Project. Neuroimage 80:105–124
Preti MG, Van De Ville D (2019) Decoupling of brain function from structure reveals regional behavioral specialization in humans [no. 1]. Nat Commun 10:4747
Liu TT, Nalci A, Falahpour M (2017) The global signal in fMRI: nuisance or information? Neuroimage 150:213–229
Murphy K, Fox MD (2017) Towards a consensus regarding global signal regression for resting state functional connectivity MRI. Neuroimage 154:169–173
Cho JW, Korchmaros A, Vogelstein JT, Milham MP, Xu T (2021) Impact of concatenating fMRI data on reliability for functional connectomics. Neuroimage 226:117549
Horn A, Li N, Dembek TA, Kappel A, Boulay C, Ewert S et al (2019) Lead-DBS v2: towards a comprehensive pipeline for deep brain stimulation imaging. Neuroimage 184:293–316
Husch A, Petersen MV, Gemmar P, Goncalves J, Hertel F (2018) PaCER—a fully automated method for electrode trajectory and contact reconstruction in deep brain stimulation. NeuroImage Clin 17:80–89
Horn A, Kühn AA (2015) Lead-DBS: a toolbox for deep brain stimulation electrode localizations and visualizations. Neuroimage 107:127–135
Dembek TA, Hellerbach A, Jergas H, Eichner M, Wirths J, Dafsari HS et al (2021) DiODe v2: unambiguous and fully-automated detection of directional DBS lead orientation [no. 11]. Brain Sci 11:1450
Åström M, Samuelsson J, Roothans J, Fytagoridis A, Ryzhkov M, Nijlunsing R, Blomstedt P (2018) Prediction of electrode contacts for clinically effective deep brain stimulation in essential tremor. Stereotact Funct Neurosurg 96:281–288
Tournier J-D, Smith R, Raffelt D, Tabbara R, Dhollander T, Pietsch M et al (2019) MRtrix3: a fast, flexible and open software framework for medical image processing and visualisation. Neuroimage 202:116137
Veraart J, Novikov DS, Christiaens D, Ades-aron B, Sijbers J, Fieremans E (2016) Denoising of diffusion MRI using random matrix theory. Neuroimage 142:394–406
Kellner E, Dhital B, Kiselev VG, Reisert M (2016) Gibbs-ringing artifact removal based on local subvoxel-shifts. Magn Reson Med 76:1574–1581
Schilling KG, Blaber J, Huo Y, Newton A, Hansen C, Nath V et al (2019) Synthesized b0 for diffusion distortion correction (Synb0-DisCo). Magn Reson Imaging 64:62–70
Andersson JLR, Sotiropoulos SN (2016) An integrated approach to correction for off-resonance effects and subject movement in diffusion MR imaging. Neuroimage 125:1063–1078
Dhollander T, Raffelt D, Connelly A (2016) Unsupervised 3-tissue response function estimation from single-shell or multi-shell diffusion MR data without a co-registered T1 image. In: ISMRM workshop on breaking the barriers of diffusion MRI, p 5
Jeurissen B, Tournier J-D, Dhollander T, Connelly A, Sijbers J (2014) Multi-tissue constrained spherical deconvolution for improved analysis of multi-shell diffusion MRI data. Neuroimage 103:411–426
Raffelt D, Dhollander T, Tournier J-D, Tabbara R, Smith RE, Pierre E, Connelly A (2017) Bias field correction and intensity normalisation for quantitative analysis of apparent fibre density. In: Proceedings of the international society for magnetic resonance in medicine, vol 25, p 3541
Smith RE, Tournier J-D, Calamante F, Connelly A (2012) Anatomically-constrained tractography: improved diffusion MRI streamlines tractography through effective use of anatomical information. Neuroimage 62:1924–1938
Smith RE, Tournier J-D, Calamante F, Connelly A (2015) SIFT2: enabling dense quantitative assessment of brain white matter connectivity using streamlines tractography. Neuroimage 119:338–351
Smith R (2022) Quantitative streamlines tractography: methods and inter-subject normalisation. Aperture Neuro 2:1–23
Zhang F, Daducci A, He Y, Schiavi S, Seguin C, Smith R et al (2022) Quantitative mapping of the brain’s structural connectivity using diffusion MRI tractography: a review. Neuroimage 249:118870
Horn A, Reich M, Vorwerk J, Li N, Wenzel G, Fang Q et al (2017) Connectivity predicts deep brain stimulation outcome in Parkinson disease. Ann Neurol 82:67–78
Pijnenburg R, Scholtens LH, Ardesch DJ, de Lange SC, Wei Y, van den Heuvel MP (2021) Myelo- and cytoarchitectonic microstructural and functional human cortical atlases reconstructed in common MRI space. Neuroimage 239:118274
Nichols TE, Holmes AP (2002) Nonparametric permutation tests for functional neuroimaging: a primer with examples. Hum Brain Mapp 15:1–25
Vergani F, Martino J, Morris C, Attems J, Ashkan K, Dell’Acqua F (2016) Anatomic connections of the subgenual cingulate region. Neurosurgery 79:465–472
Johansen-Berg H, Gutman DA, Behrens TEJ, Matthews PM, Rushworth MFS, Katz E et al (2008) Anatomical connectivity of the subgenual cingulate region targeted with deep brain stimulation for treatment-resistant depression. Cereb Cortex 18:1374–1383
Mayberg HS, Liotti M, Brannan SK, McGinnis S, Mahurin RK, Jerabek PA et al (1999) Reciprocal limbic-cortical function and negative mood: converging PET findings in depression and normal sadness. Am J Psychiatry 156:675–682
Berman MG, Peltier S, Nee DE, Kross E, Deldin PJ, Jonides J (2011) Depression, rumination and the default network. Soc Cogn Affect Neurosci 6:548–555
Mayberg HS (1997) Limbic-cortical dysregulation: a proposed model of depression. J Neuropsychiatry Clin Neurosci 9:471–481
Mayberg HS et al (2009) Targeted electrode-based modulation of neural circuits for depression. J Clin Investig 119:717–725
Kito S, Fujita K, Koga Y (2008) Regional cerebral blood flow changes after low-frequency transcranial magnetic stimulation of the right dorsolateral prefrontal cortex in treatment-resistant depression. Neuropsychobiology 58:29–36
Holtzheimer PE, Husain MM, Lisanby SH, Taylor SF, Whitworth LA, McClintock S et al (2017) Subcallosal cingulate deep brain stimulation for treatment-resistant depression: a multisite, randomised, sham-controlled trial. Lancet Psychiatry 4:839–849
Riva-Posse P, Choi KS, Holtzheimer PE, McIntyre CC, Gross RE, Chaturvedi A et al (2014) Defining critical white matter pathways mediating successful subcallosal cingulate deep brain stimulation for treatment-resistant depression. Biol Psychiatry 76:963–969
Song J (2023) Amygdala activity and amygdala-hippocampus connectivity: metabolic diseases, dementia, and neuropsychiatric issues. Biomed Pharmacother 162:114647
Morishita T, Fayad SM, Higuchi M, Nestor KA, Foote KD (2014) Deep brain stimulation for treatment-resistant depression: systematic review of clinical outcomes. Neurotherapeutics 11:475–484
Castrén E (2005) Is mood chemistry? Nat Rev Neurosci 6:241–246
O’Reardon JP, Solvason HB, Janicak PG, Sampson S, Isenberg KE, Nahas Z et al (2007) Efficacy and safety of transcranial magnetic stimulation in the acute treatment of major depression: a multisite randomized controlled trial. Biol Psychiatry 62:1208–1216
Cohen MX, Axmacher N, Lenartz D, Elger CE, Sturm V, Schlaepfer TE (2009) Neuroelectric signatures of reward learning and decision-making in the human nucleus accumbens. Neuropsychopharmacology 34:1649–1658
Bewernick BH, Kayser S, Gippert SM, Switala C, Coenen VA, Schlaepfer TE (2017) Deep brain stimulation to the medial forebrain bundle for depression-long-term outcomes and a novel data analysis strategy. Brain Stimul 10:664–671
Fenoy AJ, Schulz PE, Selvaraj S, Burrows CL, Zunta-Soares G, Durkin K et al (2018) A longitudinal study on deep brain stimulation of the medial forebrain bundle for treatment-resistant depression [no. 1]. Transl Psychiatry 8:1–11
Lozano AM, Mayberg HS, Giacobbe P, Hamani C, Craddock RC, Kennedy SH (2008) Subcallosal cingulate gyrus deep brain stimulation for treatment-resistant depression. Biol Psychiatry 64:461–467
Lozano AM, Giacobbe P, Hamani C, Rizvi SJ, Kennedy SH, Kolivakis TT et al (2012) A multicenter pilot study of subcallosal cingulate area deep brain stimulation for treatment-resistant depression. J Neurosurg 116:315–322
Jiménez F, Velasco F, Salin-Pascual R, Hernández JA, Velasco M, Criales JL, Nicolini H (2005) A patient with a resistant major depression disorder treated with deep brain stimulation in the inferior thalamic peduncle. Neurosurgery 57:585–593
Raymaekers S, Luyten L, Bervoets C, Gabriëls L, Nuttin B (2017) Deep brain stimulation for treatment-resistant major depressive disorder: a comparison of two targets and long-term follow-up. Transl Psychiatry 7:e1251–e1251
Coenen VA, Schlaepfer TE, Sajonz B, Döbrössy M, Kaller CP, Urbach H, Reisert M (2020) Tractographic description of major subcortical projection pathways passing the anterior limb of the internal capsule. Corticopetal organization of networks relevant for psychiatric disorders. NeuroImage Clin 25:102165
Accolla EA, Aust S, Merkl A, Schneider G-H, Kühn AA, Bajbouj M, Draganski B (2016) Deep brain stimulation of the posterior gyrus rectus region for treatment resistant depression. J Affect Disord 194:33–37
Kirsch I, Deacon BJ, Huedo-Medina TB, Scoboria A, Moore TJ, Johnson BT (2008) Initial severity and antidepressant benefits: a meta-analysis of data submitted to the Food and Drug Administration. PLoS Med 5:e45
Wang Q, Akram H, Muthuraman M, Gonzalez-Escamilla G, Sheth SA, Oxenford S et al (2021) Normative vs. patient-specific brain connectivity in deep brain stimulation. Neuroimage 224:117307
Scangos KW, Khambhati AN, Daly PM, Makhoul GS, Sugrue LP, Zamanian H et al (2021) Closed-loop neuromodulation in an individual with treatment-resistant depression. Nat Med 27:1696–1700
Acknowledgements
This work was supported by the Swiss National Science Foundation (grant number 186142). Preliminary results from this study were presented as a poster at the conference OptoDBS 2022. We thank the patients for providing their consent. We also thank Florian Weiss, Christoph Stucki and Michael Strehlen for performing some of the clinical assessments.
Funding
Open access funding provided by University of Bern.
Author information
Authors and Affiliations
Corresponding author
Ethics declarations
Conflict of interest
Author AN received consultant fees from Medtronic, not relevant to the submitted work. Author ID received research and travel grants from Boston Scientific, not relevant to the submitted work. Author MGP was supported by the CIBM Center for Biomedical Imaging, a Swiss research center of excellence founded and supported by Lausanne University Hospital (CHUV), University of Lausanne (UNIL), Ecole Polytechnique Fédérale de Lausanne (EPFL), University of Geneva (UNIGE) and Geneva University Hospitals (HUG), all not relevant to the submitted work. Author DVDV received funding from the Swiss National Science Foundation (Grants 205321-207493, CRSII5_209470, CRSII5_180319), Horizon Europe/SERI (grant MICROVASC), PHRT Pioneer Imaging Project, Bertarelli Foundation, Boninchi Foundation, Wyss Center Geneva, Dandelion Science Inc., as well as honoraria from Elsevier Inc., all not relevant to the submitted work. Author CP is co-founder of Aleva Neurotherapeutics SA and received consultant fees from Boston Scientific and Abbott, not relevant to the submitted work. Author SW received funding from the Swiss National Science Foundation (Grants 182469 and 184717), the Novartis Foundation for Biomedical Research, the Swiss Health Promotion Foundation and the National Institutes of Health (R01 MH 118741), as well honoraria from Lundbeck, Mepha, and Neurolite, all not relevant to the submitted work. The remaining authors declare that the research was conducted in the absence of any commercial or financial relationships that could be construed as a potential conflict of interest.
Supplementary Information
Below is the link to the electronic supplementary material.
Rights and permissions
Open Access This article is licensed under a Creative Commons Attribution 4.0 International License, which permits use, sharing, adaptation, distribution and reproduction in any medium or format, as long as you give appropriate credit to the original author(s) and the source, provide a link to the Creative Commons licence, and indicate if changes were made. The images or other third party material in this article are included in the article's Creative Commons licence, unless indicated otherwise in a credit line to the material. If material is not included in the article's Creative Commons licence and your intended use is not permitted by statutory regulation or exceeds the permitted use, you will need to obtain permission directly from the copyright holder. To view a copy of this licence, visit http://creativecommons.org/licenses/by/4.0/.
About this article
Cite this article
Leserri, S., Segura-Amil, A., Nowacki, A. et al. Linking connectivity of deep brain stimulation of nucleus accumbens area with clinical depression improvements: a retrospective longitudinal case series. Eur Arch Psychiatry Clin Neurosci 274, 685–696 (2024). https://doi.org/10.1007/s00406-023-01683-x
Received:
Accepted:
Published:
Issue Date:
DOI: https://doi.org/10.1007/s00406-023-01683-x